In recent years, clustering quality measures have been calculated in cluster analysis, and many academic societies now require that papers include clustering quality measures as a criterion for adopting a particular clustering. To be honest, I have mixed feelings about this. Because I feel like the purpose of cluster analysis has been lost. Cluster analysis is an exploratory method for finding “useful” clusters within the problem user is working on, not for determining an objective classification method.
On the calculation, cluster analysis is a method of extracting clusters of data points based on their mutual distance relationship or density distribution in a multidimensional space. It’s just like looking at clouds floating in the sky. Like clouds, data clustering is very fuzzy. Just as there is little point in asking how many clouds there are, there is little point in determining the number of clusters.
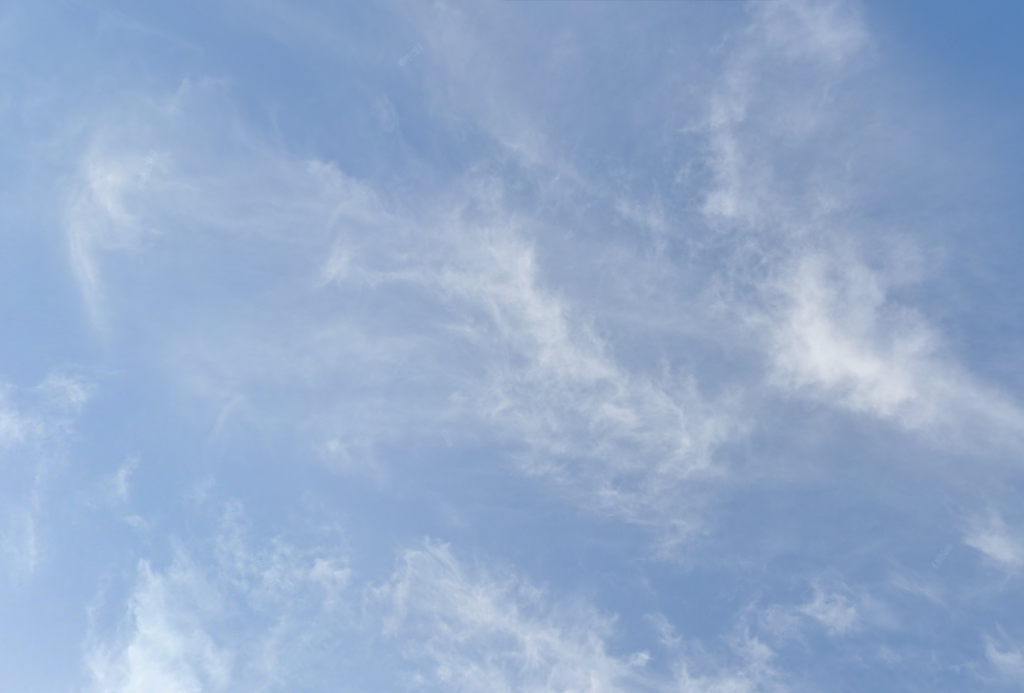
Sequentially merging smaller clusters increases the within-cluster variance. The clustering quality measure, for example, finds merging with a large growth rate and gives a higher quality measure to the clustering before merging. It helps find more “natural” clustering. However, even if a specific number of clusters is determined, it is not a mistake to adopt a different number of clusters.
For example, if you are developing a new product and want to establish target consumer personas for that product, cluster analysis can be helpful. If there are many competing products in the market and very tight differentiation is required, it makes sense to adopt a clustering that is smaller than the default clustering. In most cases, the default clustering only indicates broad categories of products.
The characteristics of each cluster must be analyzed to determine which consumers to target and what products to offer. This is called profile analysis. Cluster analysis cannot be completed with data clustering alone; it must be integrated with profile analysis. In other words, it can be called a “concept” analysis. In traditional philosophy, concepts are defined by “intension” and “extention”. Intension refers to the common properties of a certain concept, and extension refers to the examples the concept includes. There is no contradiction between the way we “develop product concepts” in business and the concepts we say in philosophy.
It is no exaggeration to say that SOM is a tool for expressing concepts inherent in data. The combination of SOM and cluster analysis is a very powerful conceptual analysis tool.
In order to delve deeper into the essence of cluster analysis, next time I will discuss the “ugly duckling theorem.”
コメントを残す